Study: Higher “possession stats” does not correlate to more winning in hockey
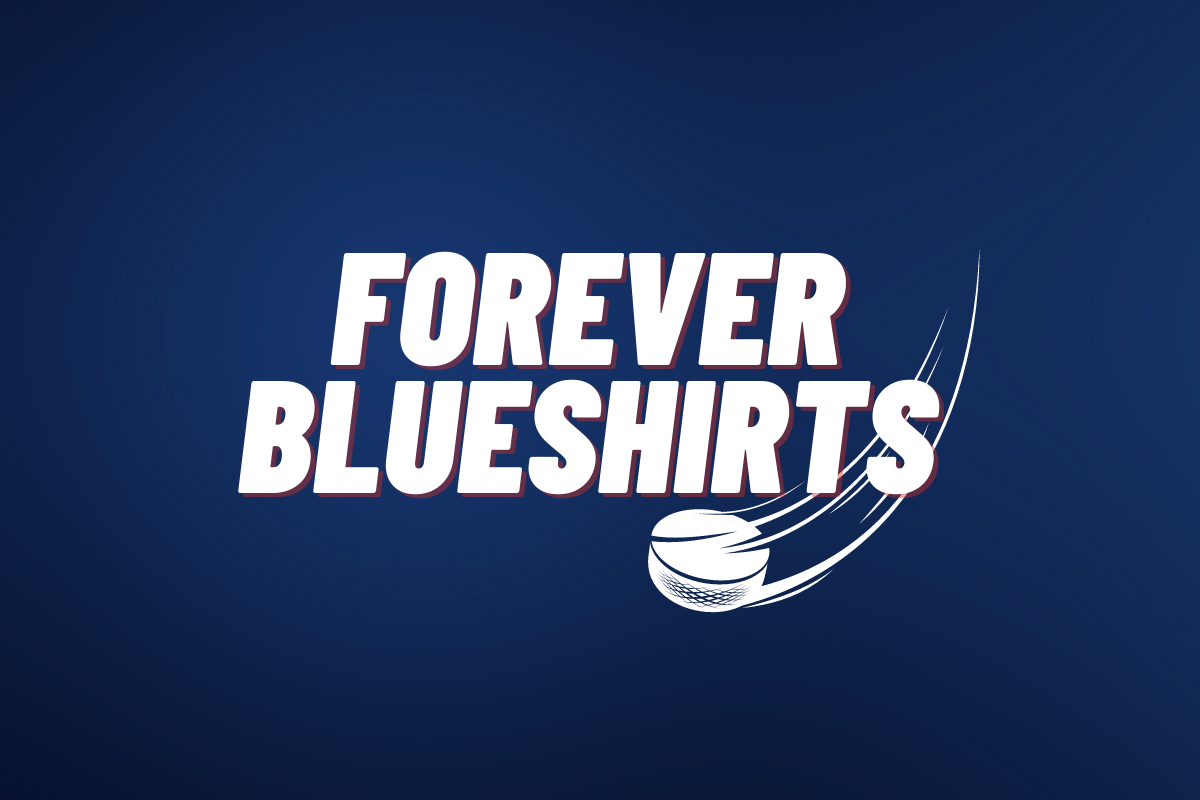
Over the last two years, I’ve pretty much bumped heads with many a person about advanced analytics in hockey. My issue isn’t necessarily the stats themselves, because I believe they provide insight and a fresh perspective on sometimes very hard to distinguish issues on the ice.
The fight is usually when I see these stats being used in small sample sizes or tweaked to make points on players like Dan Girardi (who apparently is the worst defenseman on Earth) and Dan Boyle (who although scratched twice and committed some horrendous turnovers is way better). To make matters worse are usually the people who are putting forth the narrative and how difficult they can be to deal with.
Now even though most admit these stats are flawed, they will dig in their heels and bury you if you so much as criticize or question the validity of its use and resulting hypothesis.
I make no bones about it, I’m an old school guy and rely heavily on what I’m seeing on the ice for the most part. Then take what I’ve learned from watching, playing and coaching over thousands of hockey games to make my assessment. And yes, I even check out War-On-Ice and Stats.HockeyAnalysis too. Yet still, I find more reasons to challenge the information instead of buying in.
Which leads me to this piece. One of the most common arguments about these stats is that they are great predictive indicators. Let’s focus on Corsi and Fenwick here and the notion that teams that are more proficient in these puck possession stats will over time will win more games.
For more on Corsi and Fenwick read here.
I often argue that, there are so many things going on in a game and that relying on a stat that gives a team/player a +1 for saucing one in from the blue-line into a goalie’s chest is suspect. I believe winning consistently is about talent, and more importantly adhering to a system that fits that talent. The fact that you may have good possession numbers is more a byproduct of that.
However, to be fair and to see if the statement that “good possession numbers leads to more wins” is true, I decided to put it to the test. So here’s what I did.
First, I looked to last year and entered in the CF%, FF% and wins of every team in the NHL. I gathered that info from the two sites mentioned above. Then I used a linear regression tool to see if two variables (CF or FF and Wins) had any correlation. If you haven’t taken a stats class this could go over your head, but I will try and make it understandable. Here are the results:
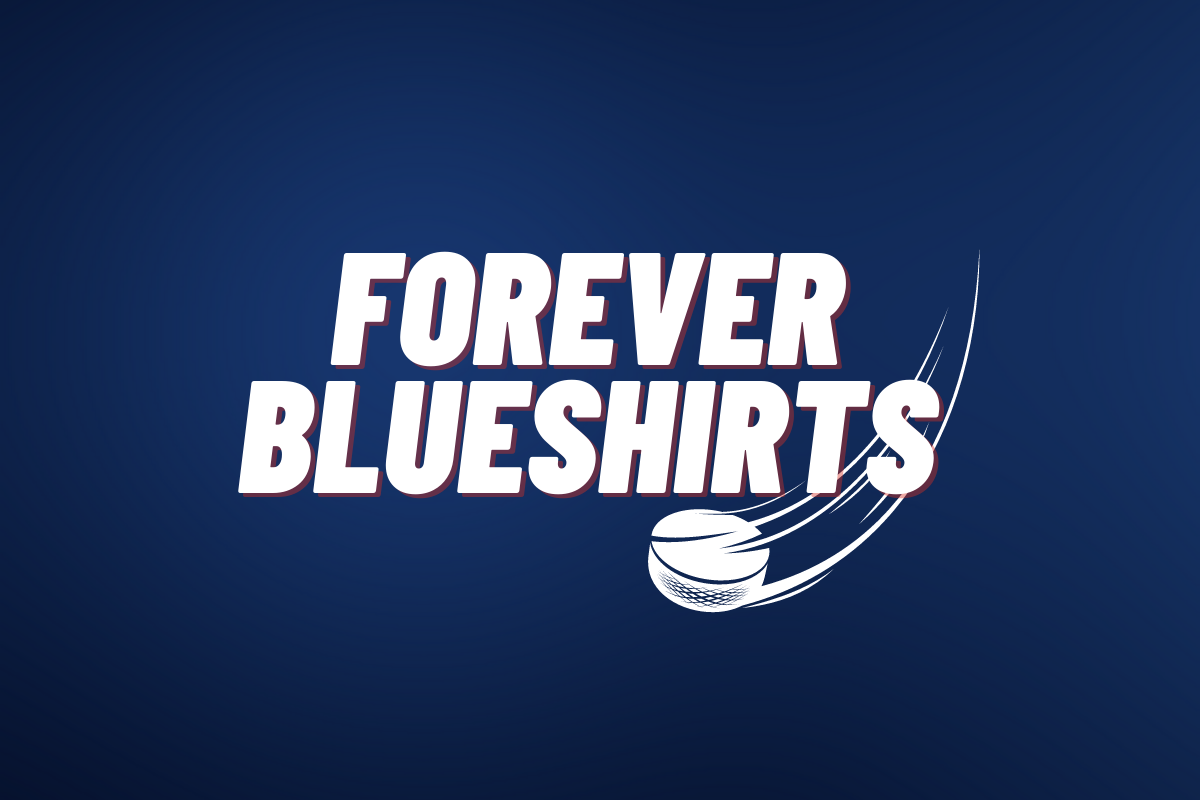
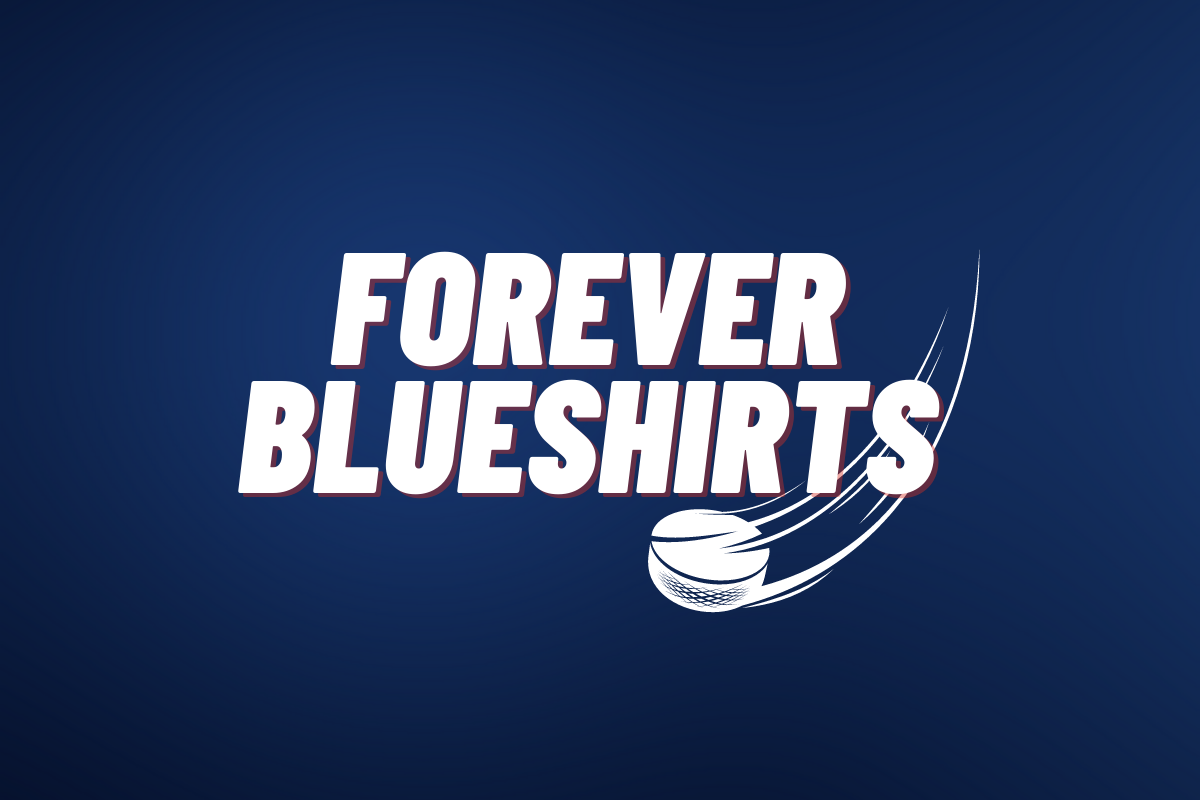
So what am I looking at you ask? That line and the blue dots. If there was a correlation between a higher CF% or FF% and more wins, you’d see a lot more blue dots on or very close to that line.
The real key here is the r2 number. The closer to 1 that is, the more correlation can be made between the two variables. Here’s a clearer explanation for you.
[su_quote cite=”The MiniTab Blog” url=”https://blog.minitab.com/blog/adventures-in-statistics/regression-analysis-how-do-i-interpret-r-squared-and-assess-the-goodness-of-fit”]R-squared is a statistical measure of how close the data are to the fitted regression line. It is also known as the coefficient of determination, or the coefficient of multiple determination for multiple regression. The definition of R-squared is fairly straight-forward; it is the percentage of the response variable variation that is explained by a linear model. Or: R-squared = Explained variation / Total variation R-squared is always between 0 and 100%: 0% indicates that the model explains none of the variability of the response data around its mean. 100% indicates that the model explains all the variability of the response data around its mean.[/su_quote]
So the CF regression model accounts for about 22% of the variance and the FF regression model about 30%. As stated above, the more variance the regression model can account for, the more the blue dots come closer to the line.
The above two models indicates to me that there is no correlation that can be made by having higher “possession stats” leading to more wins.
Now, I wouldn’t be doing a good job if I just stopped after one year. Someone would certainly say that season could be an outlier. An outlier is basically something that says, “I can’t explain it. It doesn’t help my argument, so I’m throwing it away.”
To finally answer the question of “better possession stats means more wins”, I compiled 4 years of data from last year to 2011-12. All 30 teams CF and wins were tallied up and entered into the model. Here’s the results.
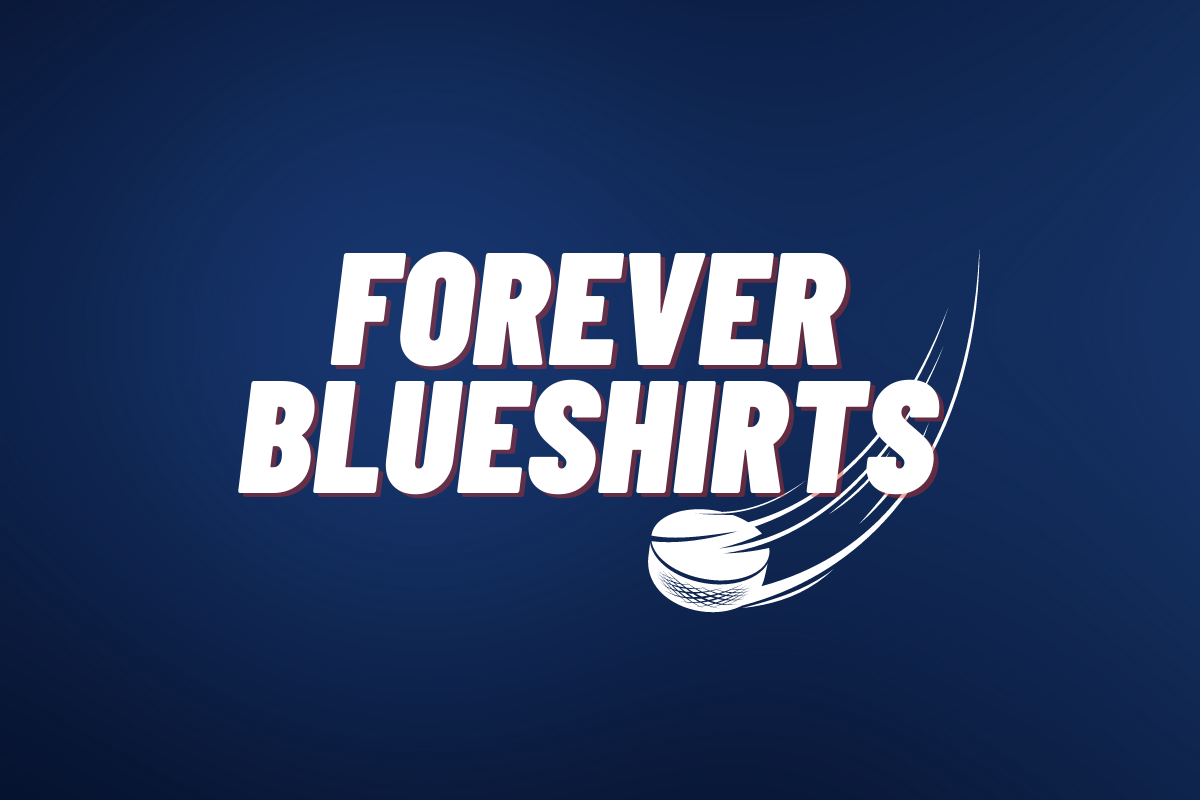
Increasing the sample size did improve the r2, but it still only explains 35% of the variance. The conclusion once again is that there is no correlation.
So there you have it, the statement that better corsi/fenwick stats means more wins is inaccurate. While they do provide a different way to look at how a team may be playing, it can’t be directly linked to winning and losing hockey games.
In closing, I’m not saying to throw out advanced stats in hockey. Matter of fact, I think they need to continue and obviously improve. However, that will never happen if critics like myself aren’t allowed to challenge the validity or use of these stats.
Please sound off in the comments section below.
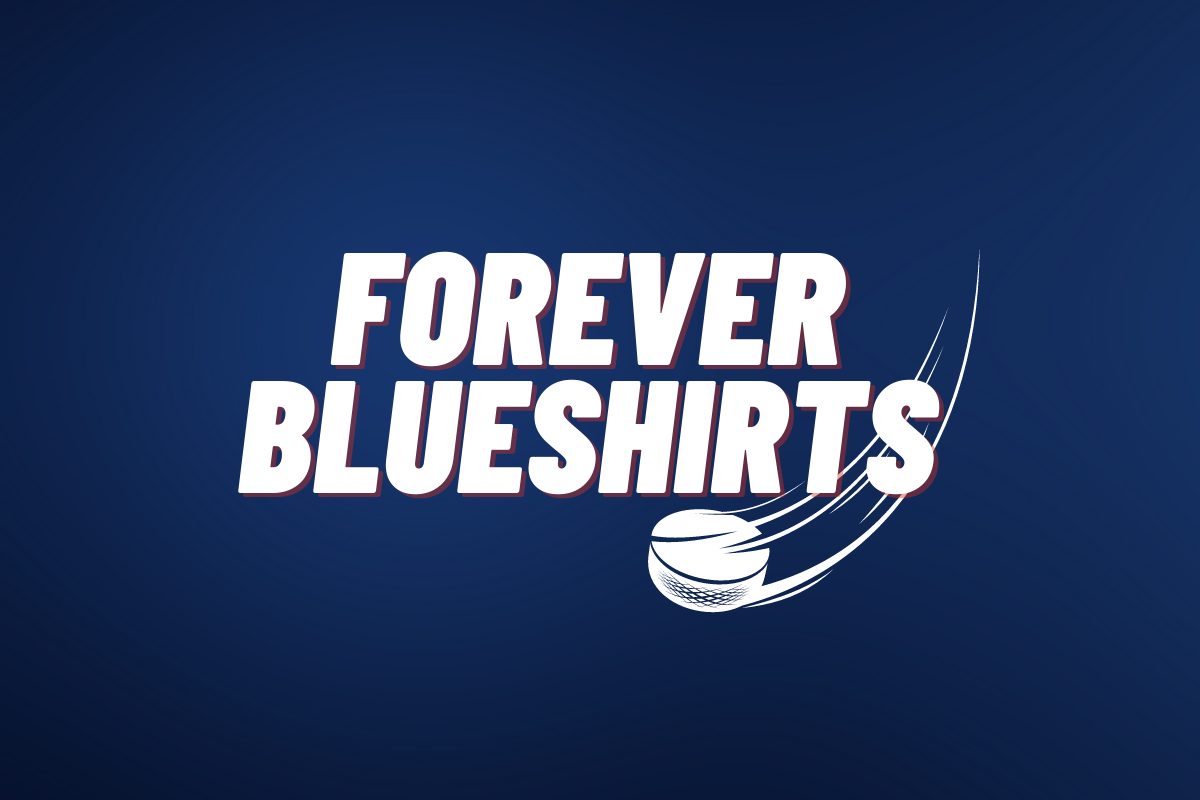
More About:New York Rangers Analysis New York Rangers News NHL News and Rumors